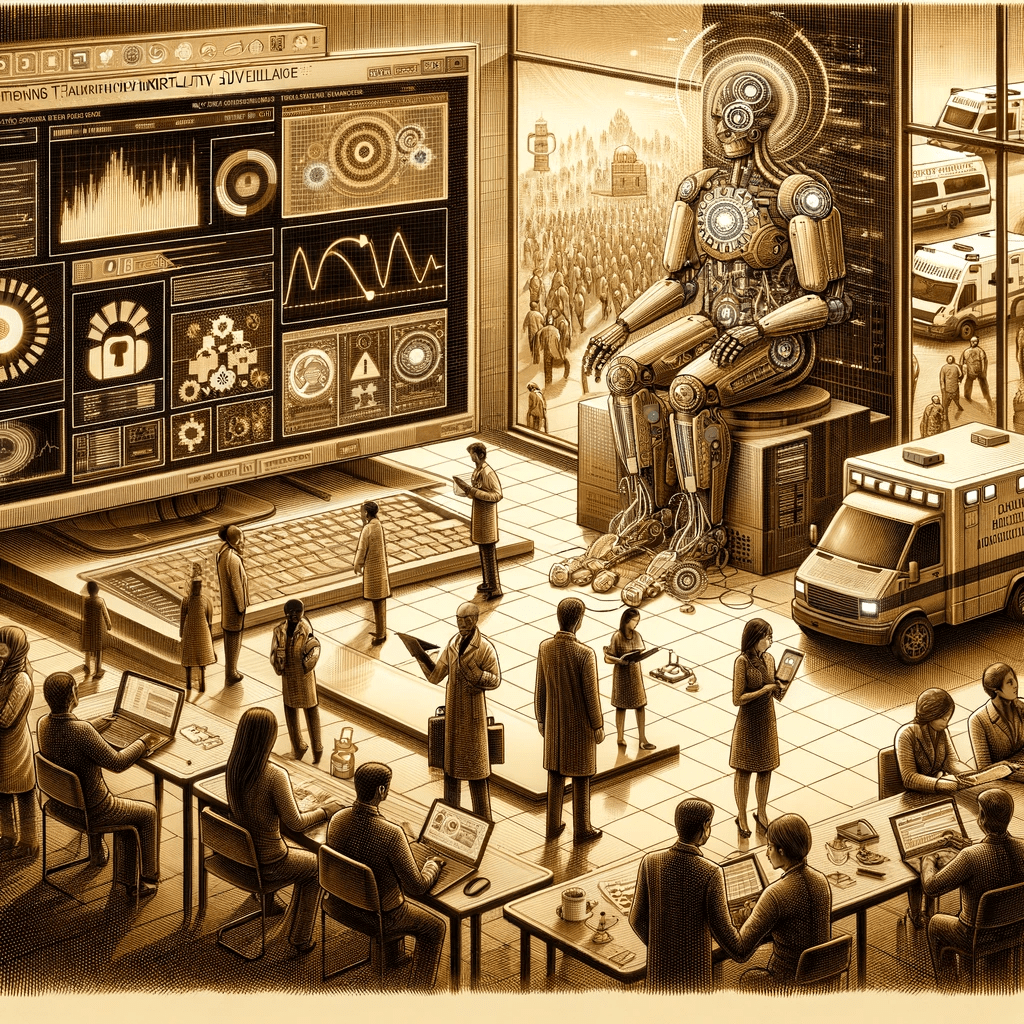
With the emergence of artificial intelligence and the development of computer systems capable of performing tasks that typically require human intelligence, this opens new opportunities in terms of mortality surveillance.
Data Analysis: Analyzing data is regularly done through manual labor although through implementing artificial intelligence, machine learning algorithms can help process that data. Algorithms created would be able to process the data at faster rates and more efficiently than ever, making responses to humanitarian crises quicker than ever before. Machine-learning methods can also provide a variety of solutions through efficiently analyzing thousands of potential predictors using data-adapting identification of intricate patterns.
Data Availability: Privacy concerns are often linked to mortality surveillance and accessing death records. Many individuals may choose not to record a certain death for privacy reasons or may remove it. Data collectors can then have access to death data that can be analyzed with algorithms that withhold people’s private information.
Potential Pitfalls: With implementing common algorithms on a variety of information the data themselves can be inherently biased. If the input data used to train algorithms lack diversity or reflect structural biases, output models will not generalize across populations. For example, because of systematic racism, black people in the United States generate less health care costs than white people. So the algorithm generating which group of people would benefit most from additional resources for healthcare would inaccurately suggest that white people would receive the most benefits.